Explanable Artificial Intelligence
Black Box system’s model provides Optimization without Justification. Predictive Mobility is using Explainable Artificial Intelligence (XAI) to make our Results Transparent, Interpretable, Reproducible, re-Traceable, re-Enactive, and most of all Comprehensible by everyone!
Once upon a Time in Cyberworld
It all started from the brilliant intellect of a group of three; one mathematician, a neurophysiologist, and a young engineer trying to develop automatic range finders for antiaircraft guns by predicting the trajectory of an airplane by taking into account the elements of past trajectories. Wiener, Bigelow, and Rosenblueth discovered during the World War II the closed loop of information necessary to correct any action, the negative feedback loop, and they generalized this discovery in terms of the human organism. Through this, Cybernetics (Cy) was born.
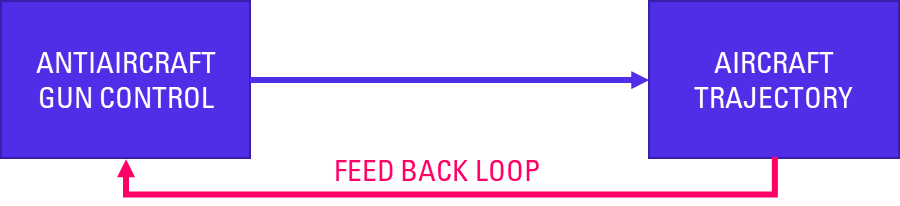
The word Cybernetics was reinvented by Norbert Wiener in 1948 from the Greek “Kubernetes”, pilot, or rudder. The word was first used by Plato in the sense of the art of steering or the art of government. Cybernetics is the science of feedback, information that travels from a system through its environment and back to the system. A feedback system is said to have a goal, such as maintaining the level of a variable (e.g., water volume, temperature, direction, or speed). Feedback reports the difference between the current state and the goal, and the system acts to correct differences. Cybernetics is the foundation of any Artificial Intelligence (AI) research, even if nowadays there are some major differences that need to be explained to understand the different approach between Cybernetics and AI.
Cybernetics and Artificial Intelligence
Cybernetics and artificial intelligence are often considered the same thing, with cybernetics having something to do with creating intelligent cyborgs and robots. In reality, cybernetics and AI are different ways of thinking about intelligent systems or systems that can act towards reaching a goal. AI is primarily concerned with making computers mimic intelligent behavior based on stored representations of the world. Cybernetics more broadly encompass the study of how systems regulate themselves and act towards goals based on feedback from the environment.
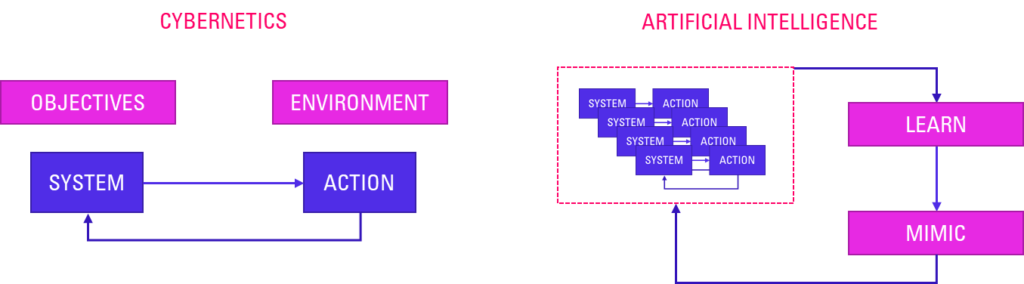
The recent upsurge in Artificial Intelligence has focused mainly on one specific area of AI: machine learning, and especially deep learning. The challenge is that these systems leave the human user out of the loop, using plenty of algorithms that are black boxes. An analyst can see the inputs as raw data and the assumptions and the outputs as optimal results or forecasts. But he will never receive a human-friendly explanation on the rationale behind the results of the AI process. This is a true challenge when you need to take a specific business decision, such as what fares should be distributed on a specific market, or what is the demand potential of a new route. Knowing the results without understanding how you get there after you validate the market data is not sufficient. Because you are engaging your responsibility in recommending some adjustments to the network of your airline or modifying the pricing structure to be applied against your competitors. Without Why; What is meaningless.
Why using Explanable Artificial Intelligence?
AI and XAI diverge from a structural process more than the terminology might let us guess, and the latest is also incorporating key Cybernetics elements, as described in the chart below.

The key conceptual change with Explanable Artificial Intelligence is that the XAI model not only has the capacity of giving us a forecast or optimization, but also of explaining why it computes that result. The new Explanation Interface displays additional information that can give the user some insights into why such forecast or optimization was made. It is vastly different from the AI process when the user simply gives the model an input, and he/she gets a result that he/she should trust. Is this possible without knowing what is happening under the hood, and without any further explanation? Our response is no, and for several reasons:
- Understanding what happens when AI models compute results help speed up the widespread adoption of these systems by the end users;
- It makes users become an integrated part of the optimization process, along with the technology, rather than being a spectator of it;
- The user can incorporate his specific market knowledge, to deliver a customized and more accurate result versus AI and Machine Learning;
- Explainable models help the users make better use of the outputs such models give, making them have even more positive impact in their business and a more efficient decision making by reverse engineering the process leading to the results.
Practical Benefits for your Decision Making
Across Predictive Mobility’s portfolio of solutions, and within each module such as pricing, revenue management, route development and network optimization, Explanable Artificial Intelligence is widely used. The obvious benefit is that our users are at the driving seats of the various processes XAI uses, incorporating to the model their markets experience. Because regardless of how sophisticated our models are, user’s expertise is as important, and help build trust in the results, intermediate and final.
The second benefit is a huge improvement in the results’ accuracy, supporting a better decision making, less risk of errors, and ultimately increasing your company’s revenue and profitability. Finally, it improves the overall robustness of our application and its optimization models, forecasts, and system recommendations.
With XAI, Predictive Mobility is growing your airline business through greater understanding!